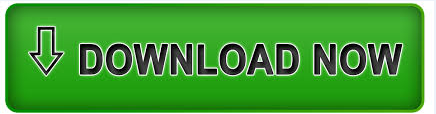
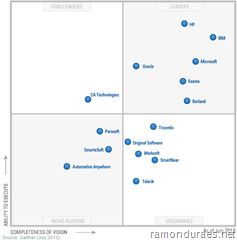
This era also saw the release of text-to-image models from Google such as Imagen (2022, using Transformer and diffusion models) and Parti (2022, using Transformers and a ViT-VQGAN model).Īnother important advancement was Latent Diffusion (2021) - a diffusion model trained within the latent space of an autoencoder. This was followed by DALL.E 2 (2022) that updated the generative part of the model to use a diffusion model. The multimodal model trend was established in with DALL.E (OpenAI), a text-to-image model based upon a discrete VAE (similar to VQ-VAE). ChatGPT (2022) is a web application and API wrapper around the latest version of GPT from OpenAI, that allows users to have natural conversations with the AI. Some open-source models were also released, such as GPT-J (2021) and GPT-NeoX (2022) by EleutherAI and BLOOM (2022) by HuggingFace. A flurry of other large language models were released to rival GPT-3, including Gopher (2021) and Chinchilla (2022) by DeepMind, LaMDA (2022) and PaLM (2022) by Google and OPT (2022) by Meta. Suddenly, diffusion models were a rival for GANs in terms of image generation quality.Īround the same time, GPT-3 (2020) was released - a 175B parameter Transformer. Two models were introduced in 2020 that would lay the foundations for all future large image generation models - DDPM and DDIM.
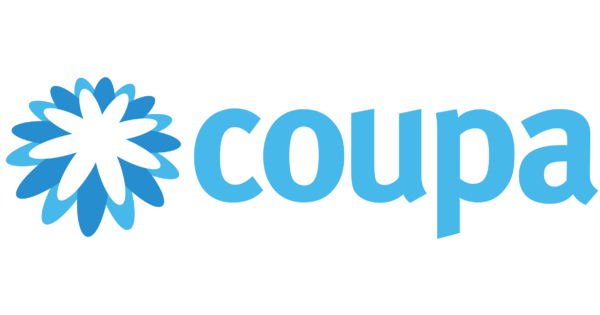
For example, VQ-GAN (2020) brought the GAN discriminator into the VQ-VAE architecture and the Vision Transformer (2020) showed how it was possible to train a Transformer to operate over images. This era saw the merging of ideas from across different generative modeling families. The following years saw progressively larger language models being built, with GPT-2 (2018, 1.5B parameters) and T5 (2019, 11B parameters) being stand-out examples. The Transformer quickly rose to prominence, with the introduction of GPT (a decoder-only Transformer). The following three years were dominated by fundamental changes to the GAN model architecture (DCGAN, 2015), loss function (Wasserstein GAN, 2017) and training process (ProGAN, 2017), as well as tackling new domains using GANs such as image-to-image translation (pix2pix, 2016 and CycleGAN, 2017) and music generation (MuseGAN, 2017). The invention of the VAE in December 2013 can perhaps be thought of as the spark that lit the generative AI touchpaper, followed by first GAN in 2014. A history of the most important generative AI models, from 2014 to 2023
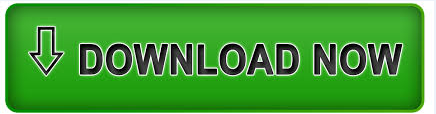